AI in healthcare: Regulatory uncertainty threatens innovation
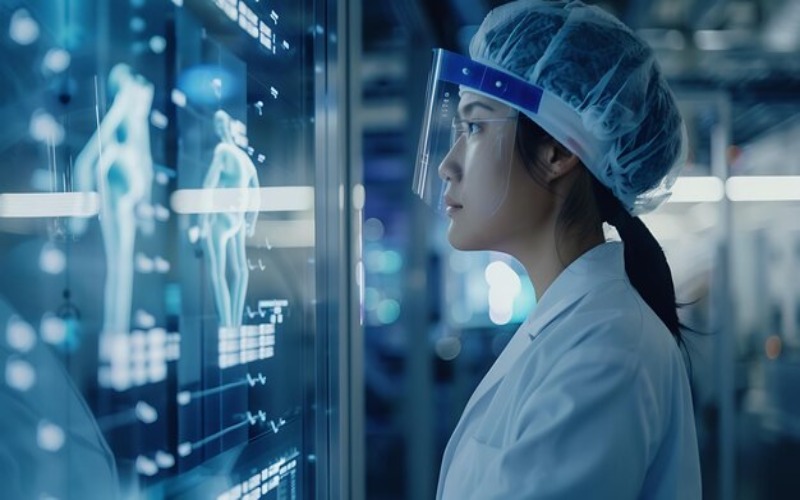
“It is not the strongest species that survives, nor the most intelligent, but the one that responds most to change. » -Charles Darwin
The healthcare industry is at the forefront of the AI revolution. The benefits of AI are easy to see in healthcare: helping patients gain health knowledge, faster R&D and faster completion of clinical trials, more efficient diagnosis of rare diseases, fewer errors in the exchange of patient data between organizations and IT systems, and time automation. consumer-intensive business processes – all fueling the utopian goals of reducing costs and risks while increasing regulatory compliance.
AI Regulations – Current Status and Gaps
The lack of clear regulations governing the use of AI in healthcare limits its progress. In general, the healthcare industry is one of the most regulated industries, with frameworks such as the FDA GxP guidelines, as summarized in 21 CFR Part 11. These regulations encourage best practices, increase transparency, and make different stakeholders more responsible for managing patient health. Unfortunately, regulatory regimes have failed to keep pace with technological advancements, particularly in AI.
The problem is exacerbated by the proliferation of startups focused on applying AI to different aspects of the healthcare value chain. With the backing of angel investors and venture capital funds, more than 4,000 AI startups have launched in the past two years, and analysts expect 10,000 more to start within the years future. With so much innovation and investment, it is even more imperative to address legitimate concerns about bias in training data, hallucinations and the likelihood of incorrect results, data privacy and information security, lack of ethical standards in regions, data interoperability between regions. health systems, among others.
In the absence of federal standards, many states have proposed separate AI bills and regulations that, if enacted, would create anything but a holistic approach essential to driving industry adoption. Recently, California, one of the largest technology hubs in America, is trying to address these issues with bills SB 1047 and AB 2013, which regulate AI, which are currently on the California Governor’s desk, Gavin Newsom. SB 1047 is not only an ambitious bill designed to enforce safety standards for the development of large AI models, but it could also serve as a barometer of future AI regulation and affect the future of health care in the United States.
On the global stage, the European Union has taken the lead in setting AI regulations under the AI Act of March 2024. Under the Act, any AI system that is a Class IIa (or higher) medical device or one that uses an AI system as a safety component is designated as “high risk.” Such systems will need to comply with a range of additional requirements, many of which overlap with the current rigorous conformity assessment requirements under the EU’s MDR and IVDR. However, the AI Act does not cover the use of AI in many critical areas, such as drug discovery, clinical trial recruitment, pharmacovigilance, and member registration.
There is also a lack of clear guidance on the applicability of audit and CSV requirements (such as IQ/OQ/PQ processes) that are part of 21 CFR Part 11 for AI systems in healthcare. health.
Bias and Incorrect Datasets for Training
Another major challenge is inherent bias and incorrect information in the available data set.
One of the most visible cases highlighting this challenge is the research of Derek Driggs, an ML researcher at the University of Cambridge. Driggs built an ML model for disease diagnosis with better accuracy than doctors. However, further investigation revealed that his model was flawed because it was trained on a dataset that included scans of both lying and standing patients. Patients who were lying down were much more likely to be seriously ill. The algorithm therefore learned to identify disease risk based on the person’s position in the analysis.
This case bears an uncanny resemblance to another famous experiment where an AI model learned to determine whether images depicted wolves or huskies by searching for snow, since the original training datasets for the algorithm included a disproportionate number of images of wolves in winter instead of focusing on snow. animals and their differences.
These examples are not isolated cases. Bias and incorrect data sets are intrinsic and constant risks for every AI model. A robust AI framework would help build transparency and predictability in which patients and regulators can trust AI models.
AI-powered diagnostics, automation and drug discovery:
Many more use cases have emerged where AI is used in patient care, disease diagnosis, workflow automation, drug discovery, and many more. These use cases can mainly be categorized as areas where AI is primarily used to increase the speed and accuracy of existing working models. Let’s look at some of these use cases:
- Patient Diagnosis: AI algorithms analyze medical imaging data, such as X-rays, MRIs and CT scans, to help healthcare professionals make accurate and rapid diagnoses.
- Medical Document Transcription: Automatic Speech Recognition (ASR) technology uses advanced algorithms and machine learning models to convert spoken language into written text, providing a more efficient and accurate method for documenting medical information .
- Drug discovery and development: AI accelerates the drug discovery process by analyzing large data sets to identify potential drug candidates and predict their effectiveness.
- Administrative efficiency: AI streamlines administrative tasks, such as billing and scheduling, thereby reducing paperwork and improving overall operational efficiency within healthcare organizations.
As the number and usage of use cases increases, a fundamental paradox is at the heart here.
The current approach to health care regulation relies on predictability. Regulators like the FDA review drug and device approval applications (NDAs/BLAs/PMAs) based on safety and effectiveness data generated during controlled trials.
However, the world of AI relies on constant training with new data sets. Limiting the AI model to a controlled data set or constrained environment defeats the purpose of the machine learning capability that makes AI effective. AI models should change their answer to the same question as they learn from the slightest differences in the environment.
These two approaches are opposed. Without a clear regulatory framework, sponsors and users of the AI use case assume responsibility and brand risk. This truly is a new frontier and we need health policy breakthroughs as dramatic as AI.
Conclusion
The World Health Organization’s recent publication, which lists key regulatory considerations for artificial intelligence (AI) for health, may be a good start to untangling these complex issues. The 18 regulatory considerations addressed in the publication fall into six broad categories: documentation and transparency, risk management, intended use and validation, data quality, data privacy and protection, and engagement and collaboration.
It’s up to industry, regulators, academia, lawmakers, and patient organizations to come together to ensure AI delivers on the promise of a better (and healthier) world.
Leave a Comment